U S GOVERNMENT MOVES $2 BILLION WORTH OF BITCOIN
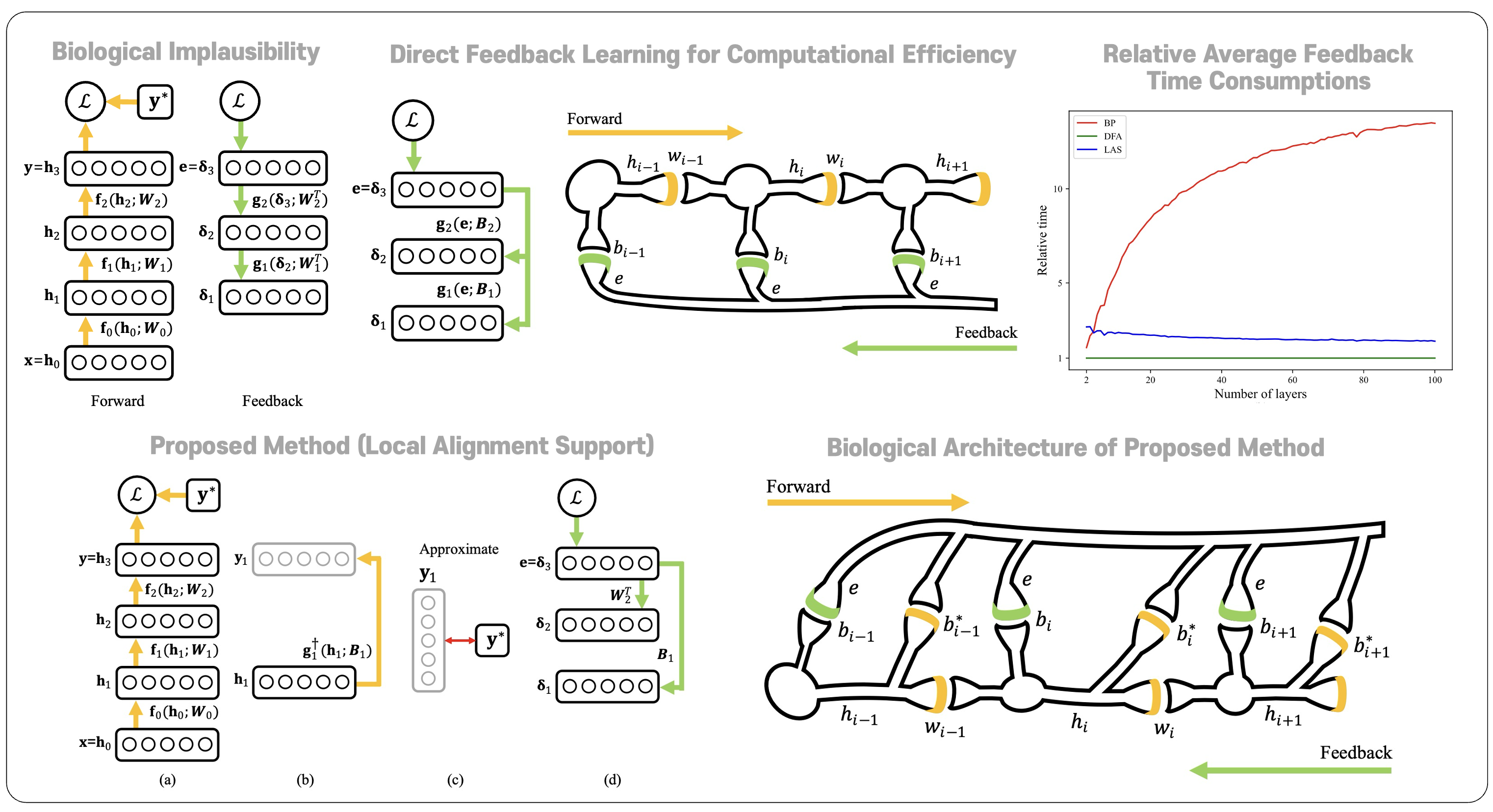
Error backpropagation led to significant advances in deep learning by solving the credit assignment problem of multi-layer perceptron. However, it suffers from a variety of biological inplausiblity and computational inefficiency. To overcome these, there has been research on learning methods that use random weights and learning methods that can be parallelized. In our laboratory, we proposed layer-wise parallel learnings method that can train the feedback synaptic weights by synchronizing between forward propagated signal and direct forward propagated signal. Currently, we are researching the various learning methods based on Predictive Coding theory.
오류 역전파 알고리즘은 심층 신경망에서 각 레이어별 오류 할당 문제를 해결하여 딥러닝의 성공에 기여하였습니다. 그러나 이러한 오류 역전파 학습법은 여러가지 생물학적 비근사성 문제와 계산 비효율성 문제를 가지고 있습니다. 이를 해결하기 위해 무작위 역방향 시냅스 가중치를 사용하는 방법, 레이어별 병렬 역전파를 수행하는 방법 등 여러가지 학습법이 제안되어 왔습니다. 저희는 신경망의 순방향 전파와 직접 순방향 전파의 신호를 동기화하는 방향으로 직접 역방향 가중치를 학습하고, 이를 통하여 신경망을 레이어별 병렬 학습하는 방법을 제안하였고, 현재는 Predictive Coding 이론에 기반한 학습 이론을 연구 중에 있습니다.